Artificial intelligence for forecasting heating and cooling demands
Overview of the status and impact of the innovation
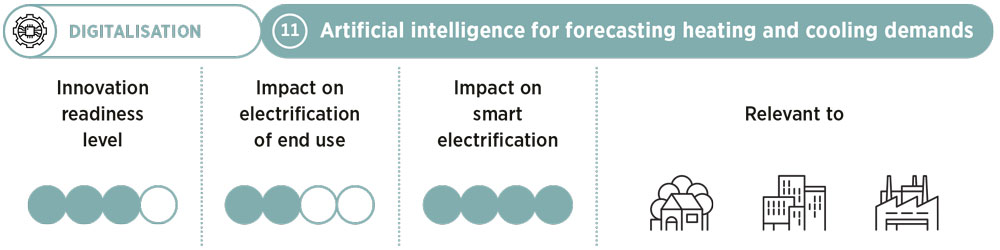
What
AI adds intelligence to the data and communications among the different components of IoT. One particularly promising use of AI is developing predictive energy consumption models for buildings that factor in the buildings’ thermal characteristics and architecture, meteorological conditions, solar irradiance, wind velocity and direction, outdoor and indoor temperatures and user behaviour. Recent research shows that novel data-driven AI- or artificial neural network–based approaches can improve the accuracy of these predictions and enable predicting short-term fluctuations, a capability essential for control applications (Bünning et al., 2020; Petrichenko et al., 2017; Saloux and Candanedo, 2018).
Why
By forecasting heating and cooling demands better, and then controlling loads to optimise their operation, AI enables greater flexibility in the energy system and facilitates increased use of variable renewable energy sources. Data-driven approaches using AI techniques such as deep learning also add significant energy savings. For example, the majority of buildings are mostly empty (more than 60% of the time), yet they maintain air temperatures of 21-23°C inside. AI methods can detect when a building is completely empty and then lower temperature setpoints in winter or raise them in summer during those periods. Further, AI can help size heating and cooling storage facilities better.
Related kits
Power to heat and cooling innovations
Innovations (35)
-
Technology and infrastructure
- 1 Low-temperature heat pumps
- 2 Hybrid heat pumps
- 3 High-temperature heat pumps
- 4 Waste heat-to-power technologies
- 5 High-temperature electricity-based applications for industry
- 6 Low-temperature thermal energy storage
- 7 Medium- and high-temperature thermal energy storage
- 8 Fourth-generation DHC systems
- 9 Fifth-generation DHC systems
- 10 Internet of Things for smart electrification
- 11 Artificial intelligence for forecasting heating and cooling demands
- 12 Blockchain for enabling transactions
- 13 Digitalisation as a flexibility enabler
-
Market design and regulation
- 14 Dynamic tariffs
- 15 Flexible power purchase agreement
- 16 Flexible power purchase agreement
- 17 Standards and certification for improved predictability of heat pump operation
- 18 Energy efficiency programmes for buildings and industry
- 19 Building codes for power-to-heat solutions
- 20 Streamlining permitting procedures for thermal infrastructure
-
System planning and operation
-
Business models
- 28 Aggregators
- 29 Distributed energy resources for heating and cooling demands
- 30 Heating and cooling as a service
- 31 Waste heat recovery from data centres
- 32 Eco-industrial parks and waste heat recovery from industrial processes
- 33 Circular energy flows in cities – booster heat pumps
- 34 Community-owned district heating and cooling
- 35 Community-owned power-to-heat assets